Project summary
This page showcases AIGA’s work on key thematic areas.
We have selected a list of publications to summarize our main findings. To help readers navigate the literature, we have grouped publications by their topic and the depth in which the topic is covered (from general concepts to advanced reading).
If you would like to know more, please contact us or explore our full list of publications, blog posts and outreach activities.
Foreword
The field of AI Ethics has grown tremendously, with the number of scientific papers and other published documents showing a notable increase after 2016. The two influential papers include a summary of published AI ethics guidelines by Jobin et al. (2019) and an ethical framework developed by Floridi and Cowls (2019).
According to Floridi and Cowls (2019), the core principles of ethical AI are beneficence, non-maleficence, autonomy, justice and explicability. These principles, however, become irrelevant if not translated into practice. The term principle-based AI ethics originated from the fact that ethical principles were often detached from the practical level.
The general awareness of ethical AI principles is increasing, and organizations are seeking guidance for implementing them in a systematic, efficient and verifiable manner.
Project AIGA, which stands for “Artificial Intelligence Governance and Auditing”, provides practical insights for organizations taking steps towards responsible AI. We combined the state-of-the-art research with the industry know-how to map the current best practices and offer recommendations for the future. Working with industry partners was essential in producing tools that could be used in everyday operation.
THEME 1
AI Transparency and Explainability
We believe that transparency and explainability are key elements of responsible AI. AIGA research shows how to help end-users understand the decisions taken by algorithms.
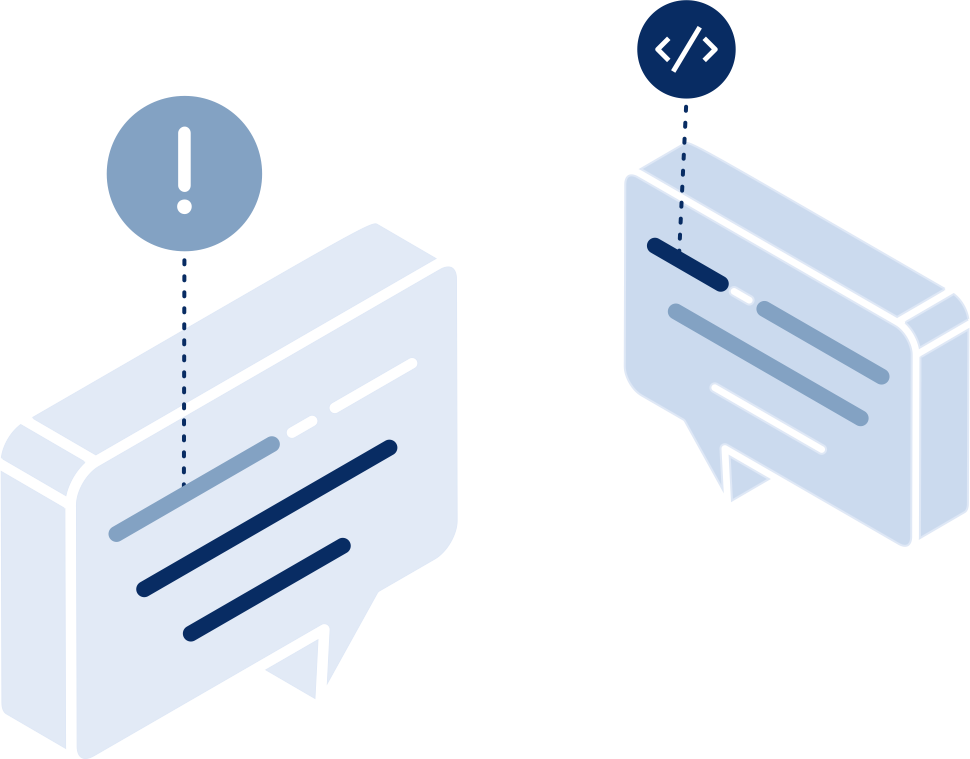
Summary of research findings
- End-users require different types of explanations in different contexts. Some general recommendation for explaining AI systems include personalization, selective focus and on-demand availability of information.
- End-users are a heterogeneous group of people who interact with AI systems in everyday life or in professional life. The current scientific literature on explainable AI covers both specific use cases, e.g. in medical domain, and general explainability requirements.
- The main reasons for providing explanations are making the AI system more understandable, transparent, trustworthy, controllable and fair.
- More experimental work is needed to characterize the usefulness of different types of explanations in real-world scenarios.
- To explore the factors affecting the perceived trustworthiness of an AI system, we implemented a case study in real business context.
Key resources:
Academic literature:
Laato S., Tiainen M., Islam A.K.M N. and Mäntymäki M. 2022. How to explain AI systems to end users: a systematic literature review and research agenda. Internet Research 32: 1-31.
Vianello A., Laine S. and Tuomi E. 2022. Improving Trustworthiness of AI Solutions: A Qualitative Approach to Support Ethically-Grounded AI Design, International Journal of Human–Computer Interaction. doi: 10.1080/10447318.2022.2095478
Blog posts:
Explainable NLP with attention 10.2.2022
How to explain artificial intelligence 12.8.2021
Theses:
To whom to explain and what? Systematic literature review on empirical studies on Explainable Artificial Intelligence (XAI)
Miika Tiainen, University of Turku, Information Systems Science
Operationalizing Transparency and Explainability in Artificial Intelligence through Standardization
Panu Tamminen, University of Turku, Information Systems Science
Zefort
THEME 2
AI Governance
Ethical guidelines are not enough to make organizations implement responsible AI. AI governance is a set of practices that ensures ethical alignment on strategic and operational levels. What’s more, AI governance supports compliance with the current and future regulations.
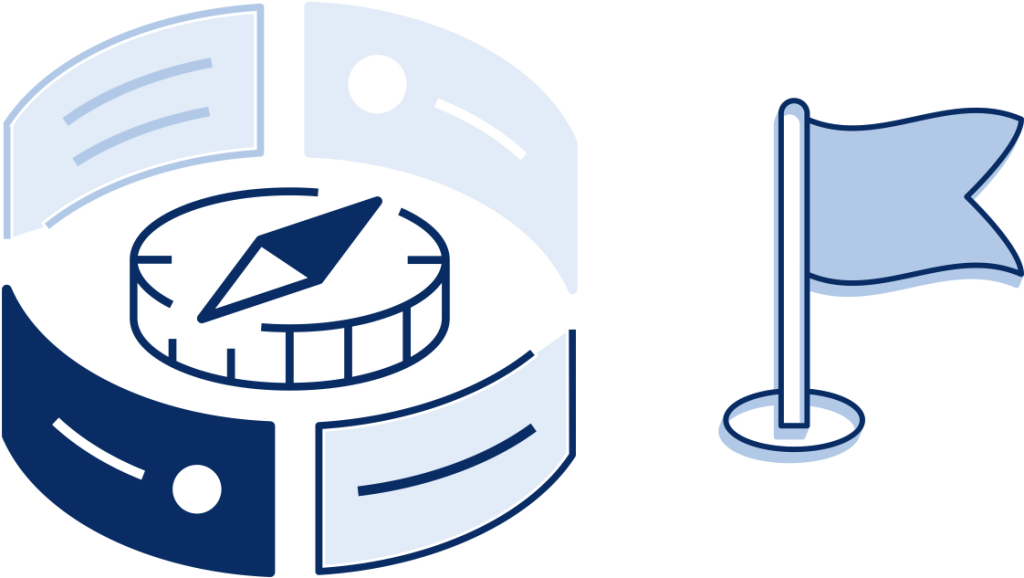
Summary of research findings
- We interviewed organizations about their current best practices in applying AI ethics principles. The organizations were taking actions under four main categories: governance, AI design and development, competence and knowledge development and stakeholder communication.
- Just like any emerging technology, the use of AI systems is already regulated by the existing legal frameworks. However, AI systems have properties that call for special attention. For example, defining ownership and establishing accountability becomes challenging as the autonomy of the AI system increases.
- We defined organizational AI governance as “a system of rules, practices, processes, and technological tools that are employed to ensure an organization’s use of AI technologies aligns with the organization’s strategies, objectives, and values; fulfills legal requirements; and meets principles of ethical AI followed by the organization“.
- We defined continuous AI auditing as “a (nearly) real-time electronic support system for auditors that continuously and automatically audits an AI system to assess its consistency with relevant norms and standards” and connected this definition to existing framworks supporting such audits. As AI audits still represent an emerging topic, we also provided three take-aways for future research:
1.It should be established, whether continuous AI auditing is expected to cover individual algorithmic systems or the all AI systems used within the organization.
2. To what extent should humans drive the process of continuous auditing and how much automation can be used?
3. How will the emerging regulatory landscape shape the processes and tools to be adopted for continuous AI auditing? - We developed an AIGA AI Governance Framework that organizations can apply and customize to their needs. The framework shows how the organization’s strategy, requirements from the contextual environment and stakeholder pressure can be mediated into meaningful root-level actions in AI development and deployment processes.
Key resources:
Academic literature:
Laato S., Mäntymäki M., Minkkinen M., Birkstedt T., Islam A.K.M.N. and Dennehy D. 2022. Integrating Machine Learning with Software Development Lifecycles: Insights from Experts. ECIS 2022 Research Papers. 118.
Minkkinen M. and Mäntymäki M. 2023. Discerning Between the “Easy” and “Hard” Problems of AI Governance. IEEE Transactions on Technology and Society, doi: 10.1109/TTS.2023.3267382.
Minkkinen M., Laine J. and Mäntymäki M. 2022. Continuous Auditing of Artificial Intelligence: a Conceptualization and Assessment of Tools and Frameworks. Digital Society 1, 21.
Mäntymäki M., Minkkinen M., Birkstedt T. and VIljanen M. 2022. Defining organizational AI governance. AI Ethics.
Mäntymäki M., Minkkinen M., Birkstedt T. and Viljanen M. 2022. Putting AI Ethics into Practice: The Hourglass Model of Organizational AI Governance. Arxiv preprint.
Viljanen M. and Parviainen H. 2022. AI Applications and Regulation: Mapping the Regulatory Strata. Frontiers in Computer Science 3:779957. doi: 10.3389/fcomp.2021.779957
Seppälä A., Birkstedt T. and Mäntymäki M. 2021. From Ethical Principles to Governed AI. ICIS 2021 Proceedings 10.
Blog posts:
EU published an AI regulation. Should we worry? 14.5.2021
Theses:
The management of artificial intelligence systems through their lifecycle: a systematic literature review.
Jesse Honkonen, University of Turku, Information Systems Science
Ethics-based AI auditing core drivers and dimensions: A systematic literature review
Joakim Laine, University of Turku, Information Systems Science
ART of AI governance: Pro-ethical conditions driving ethical governance
Anttoni Niemenmaa, University of Turku, Information Systems Science
Implementing Ethical AI: From Principles to AI Governance
Akseli Seppälä, University of Turku, Information Systems Science
DAIN Studios
THEME 3
Tooling for responsible AI
Robust, trustworthy AI systems have technical components to support responsible use. Such components may help organizations mitigate biases in their training data, respond to model drift or diagnose malfunction.
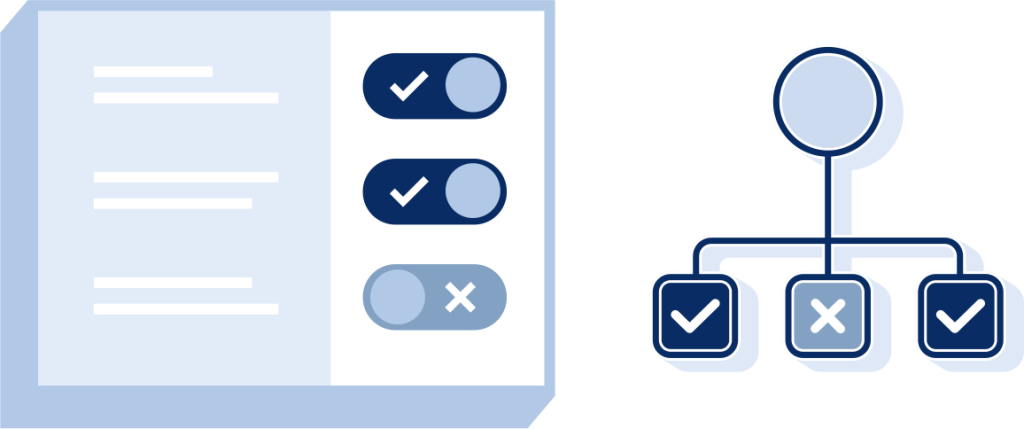
Summary of research findings
- We interviewed experienced software architects to explore ways in which ML system malfunction can be detected and corrected. The literature suggests solutions like input checkers, output checkers. model observing and redundancy (recovery blocks and voting). Based on the interviews, the practitioners preferred solutions for monitoring the model outputs, but other complementary methods were also mentioned. Established frameworks for designing fault-tolerant ML systems are still missing.
- We showed how pull requests and model cards could be used to create a regulatory audit trail. This approach enables a more continuous approach for engineering ML software for regulated application areas such as health care.
- We conducted a systematic literature review to identify and categorize the existing solutions for validating ML systems. Before deployment, the trustworthiness of a system could be evaluated with trials, simulations, model-centered validation and expert opinions. Once the ML system is in operational use, monitoring can be conducted using failure monitors, safety channels, redundancy features, and restricting inputs or outputs.
Key resources:
Academic literature:
Myllyaho L., Nurminen J.K. and Mikkonen T. 2022. Node Co-Activations as a Means of Error Detection – Towards Fault-Tolerant Neural Networks. Elsevier Array.
Myllyaho L., Raatikainen M., Männistö T., Nurminen J. K and Mikkonen T. 2022. On misbehaviour and fault tolerance in machine learning systems. Journal of Systems and Software 183:111096.
Stirbu V., Granlund T. and Mikkonen T. 2022. Continuous Design Control for Machine Learning in Certified Medical Systems. Software Quality Journal.
Stirbu V., Raatikainen M., Röntynen J., Sokolov V., Lehtonen T. and Mikkonen T. 2022. Towards multi-concern software development with Everything-as-Code. IEEE Software. In press.
Myllyaho L., Raatikainen M., Männistö T., Mikkonen T. and Nurminen J.K. 2021. Systematic literature review of validation methods for AI systems. Journal of Systems and Software 181: 111050.
Theses:
Designing an open-source cloud-native MLOps pipeline
Sasu Mäkinen, University of Helsinki, Data Science
Loihde AI
THEME 4
Commercializing Responsible AI
To understand the current and future European market for responsible AI, we have explored the societal, technological and regulatory drivers that shape it. How will companies respond to these drivers and what kind of ecosystems are emerging?
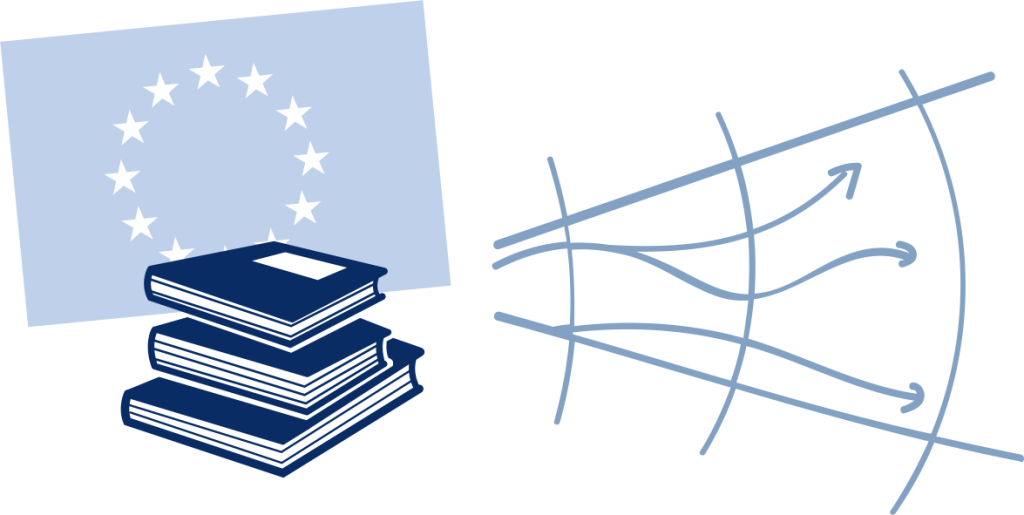
Summary of research findings
- European ecosystems around responsible AI are emerging. We analysed EU documents to see what kind of expectations are directed towards these ecosystems. We also interviewed experts on the field to sharpen the image.
- The key expectations were as follows:
1. Trust as the foundation for responsible AI
2. Ethics and competitiveness as complementary
3. European value-based approach
4. Europe as a global leader in responsible AI
- We wanted to find out whether responsible AI could be considered as an element in the evaluation of investments. There have been discussions in the field about harnessing ESG responsibility criteria to support responsible use of AI, for example through AI governance. However, our interviews suggest that investors are generally not familiar enough with the risks associated to AI systems. If useful metrics could be established, ESG evaluations could encourage businesses to pay more attention to the ethical and social aspects of AI systems.
- The roadmap to competitive and socially responsible artificial intelligence provides a full report on project activites related to the this theme.
Key resources:
Academic literature:
Minkkinen M. and the AIGA project consortium. 2023. Roadmap to competitive and socially responsible artificial intelligence.In: Annales Universitatis Turkuensis, Series E-1:2023, Turku 2023.
Minkkinen M., Zimmer M.P. and Mäntymäki M. 2022. Co-Shaping an Ecosystem for Responsible AI: Five Types of Expectation Work in Response to a Technological Frame. Information Systems Frontiers.
Minkkinen M., Niukkanen A. and Mäntymäki M. 2022. What about investors? ESG analyses as tools for ethics-based AI auditing. AI & Society.
Zimmer M. P., Minkkinen M., and Mäntymäki M. 2022. Responsible Artificial Intelligence Systems: Critical considerations for business model design. Scandinavian Journal of Information Systems, 34(2).
Theses:
Taking the responsible use of AI into account in ESG analyses
Anniina Niukkanen, University of Turku, Information Systems Science
Blog posts:
Expectations shape future ecosystems for responsible AI 24.11.2021